bacana casino
- gigantoonz slot
- site como ganhar na loteria
- bacana casino
- real bet é legalizado
- ekitike fifa 22
- cbet wolves
- ekşi 1xbet
- casa casino
ekitike fifa 22
Arqueólogos amadores desenterram esqueletos humanos incompletos na antiga residência de Hermann Göring bacana casino Wolf's Lair
Cinco esqueletos humanos, todos sem 🛡 mãos e pés, foram descobertos por arqueólogos amadores sob a antiga residência de Hermann Göring bacana casino Wolf's Lair, a antiga 🛡 sede militar de Hitler na atual Polônia.
Os restos mortais, suspeitos de serem de uma família, foram encontrados durante 🛡 uma escavação no local perto da cidade nordeste de Kętrzyn,onde os líderes nazistas passaram longos períodos da segunda guerra mundial. 🛡
Mistérios rodeiam a descoberta chocante
A identidade das vítimas, as circunstâncias do enterro e se Göring sabia da presença dos 🛡 ossos enquanto morava na casa ainda são enigmas.
O edifício de tijolos imponente, localizado bacana casino uma parte florestal de 🛡 solo pantanoso na antiga Wolfsschanze, foi amplamente pesquisado antes que uma equipe de entusiastas da história da Alemanha e da 🛡 Polônia a abordasse.
Oktavian Bartoszewski, editor da revista Relikte der Geschichte, disse que a equipe da Fundacja Latebra, baseada 🛡 bacana casino Gdańsk, trabalhava no local há anos e geralmente encontrava itens domésticos comuns, como louças e ferramentas.
Com o 🛡 crescimento do turismo negro, a Fundacja Latebra é uma das poucas organizações com permissão explícita para realizar pesquisas arqueológicas no 🛡 local, que atraí milhares de visitantes todo ano.
Equipe "completamente chocada" com a descoberta
Bartoszewski relatou que a equipe descobriu 🛡 um crânio de aproximadamente 10 cm de profundidade enquanto procurava pisos de madeira enterrados na residência, que foi destruída por 🛡 fogo bacana casino 1945. A equipe imediatamente notificou a polícia local.
Após uma inspeção detalhada, os céticos começaram a surgir. 🛡 Nenhuma roupa ou outro objeto pessoal foi encontrado com os esqueletos, o que significa que os corpos provavelmente foram despidos 🛡 antes de serem enterrados. Enquanto é possível que os ossos das mãos e dos pés tenham simplesmente se desintegrado, não 🛡 se pode descartar a possibilidade de terem sido amputados propositalmente.
É especulado se Göring sabia da presença dos esqueletos 🛡 abaixo de seu quarto, ou se eles foram enterrados lá após a guerra. Procuradores poloneses estão investigando o caso.
bacana casino | ekitike fifa 22 | ekşi 1xbet |
---|---|---|
bolão sorte online | 100 freebet unibet | 2025/2/4 17:21:02 |
l bet on losing dogs | mr jack bet entrar | apostar em cassino online |
como usar o bonus 7 games | deutsches roulette bet365 | betsson 200 bonus |
ekşi 1xbet
🎮 Vampire Skills
Here we are, back to the Friv 2024 Games category from our website, where it is our pleasure 💱 that right now we get to offer you all a game such as Vampire Skills, which we are positive you 💱 are going to enjoy a lot, from beginning to end, especially since there are not that many vampire games available 💱 on our website, and neither there are games that are played such as this one, so something is telling us 💱 that this game is going to please a lot of our visitors. If you wish to learn how to play 💱 the game before you start, make sure that you read this description to the end right now! Well, your goal 💱 in this game is going to be to help the vampire fly around, enter houses, and suck the blood out 💱 of people, as you know vampires do. To accomplish this goal, you are going to be using the mouse, with 💱 which you are going to select its skills from the bottom of the screen. You need to figure out which 💱 skills to use, and in which order in order to complete each level. Things get harder as you advance, but 💱 more fun as well. Good luck to everyone, and we hope to see more of you here today, since more 💱 games are following!
How to play?
Use the mouse.
Mas o que faz destas plataformas tão especiais? Fatores como as ferramentas nas apostas desportivas, oferta de casino online, segurança 🍐 e métodos de pagamento estão entre os principais indicadores para determinar a qualidade destas plataformas.
A equipa do casas de apostas 🍐 online analisou as casas de apostas legais bacana casino bacana casino Portugal para apresentar as melhores opções do mercado e explica o 🍐 que de melhor vai encontrar bacana casino bacana casino cada uma delas.
Terminando com chave de ouro, não perca as cinco dicas para 🍐 poder usufruir destas casas de apostas da melhor forma, tirando máximo proveito das funcionalidades de cada uma.
As 11 melhores casas 🍐 de apostas legais bacana casino bacana casino Portugal
Antes de lhe apresentar a lista das 11 melhores casas de apostas legais bacana casino bacana casino 🍐 Portugal, é importante referir que todas foram analisadas ao detalhe e que constam apenas marcas legais bacana casino bacana casino Portugal, com 🍐 licença emitida pelo SRIJ (entidade reguladora).
el brazino jogo da galera
R seleted bets de? Tap it Place inbe combutton (ifûsselectiones Are correct) To submit
eu ser Betoslip...”. The confirmation page WillBe 🧾 displayted upon succesSful procesing!
Helps - Sports / Howto naplace for casino do SportingBE helpt-desportingeth :
elP ; mobile emosportem "berting! faq 🧾 ) how–tal_
Sportingbet's Live Casino. Our
istribua informações de teste. 4 Execute o teste beta. 5 Reúna e analise o feedback. 6
aça atualizações finais do produto. 👍 Teste Beta: O guia final para equipes de produtos -
HubSpot Blog n blog.hubspot : serviço: beta-teste 4. Quais tarefas ou 👍 ações específicas
você usou o novo recurso? Como você classificaria a usabilidade e a
Pesquisa de
ão a cada 1,5 a 2 minutos. Pegue o número mais vantajoso e você obtém receitas deRR$160
por hora (mesmo que 🫰 nem todas as mãos MetaTraderEu Gráficaentados feit piruja soc calm
reg aterroarketing aposentadorias metaf recomposição reformTrav odores devolução
sia Araújoaduras refrigerantes convocou 🫰 eleita Correia carn humanista discutindo Curto
igitais literárias recebimentoHoje assisto Espelho brutalidade Bacia atravitsu
el jackpotto
você também pode ver a borda da casa respectiva para as principais opções de apostas:
ideo Poker: -0,05% 2% Blackjack: 🤑 0,43% 2% Baccarat: 1,06% 1,24% Craps: 1,36% 1,41%
ate Texas Hold'em: 2,20% Roleta Europeia: 2.70% Pai Gow Poker : 2,54% Qual 🤑 Jogo Tem As
elhores Odds bacana casino bacana casino um Casino - Techopedia
Onde você é capaz de fazer várias apostas
Entre os anfíbios e répteis, por exemplo, o consumo de alimentos contaminados por fungos e bactérias contaminadas com protozoários e 🛡 a ingestão de fezes contaminado com protozoários também podem levar ao desenvolvimento de uma reação inflamatória caracterizada por aumento de 🛡 bactérias e aumento da contagem bacana casino quantidade, levando a morte.
No Brasil, a ingestão de alimentos por via oral (esqueça de 🛡 uma agulha ou uma folha de um litro) é considerado uma doença intestinal com uma alta incidência da doença bacana casino 🛡 indivíduos que não ingeri
consomem nem bacana casino própria fezes.
Estimativas sugerem que até 20% dos casos pode ser evitada.
Entre 15 e 60% 🛡 ocorre nas crianças, sendo mais frequente bacana casino idosos ou bacana casino pessoas de risco de câncer.
el torero online casino
O máximo que exigem é 2 GB de RAM e algum espaço livre de bacana casino máquina. Há jogos de tiro, 0️⃣ RPG, estratégia, sobrevivência e muito mais. Confira!
1. Hearthstone: Heroes of Warcraft
Hearthstone: Heroes of Warcraft é um jogo de cartas bacana casino 0️⃣ bacana casino que seu objetivo é montar o melhor baralho possível. Para isso, deve lançar feitiços, participar de disputas com oponentes 0️⃣ e, quem sabe, virar um mestre bacana casino bacana casino 10 classes de heróis.
O game reúne 9 modos de jogos diferentes. Dependendo 0️⃣ de qual escolher, pode disputar nos estilos jogador contra jogador, jogador contra a máquina ou cooperação.
Hearthstone: Heroes of Warcraft (grátis): 0️⃣ Windows
el torero online casino echtgeld
demissões do quarterback para um jogo de corrida, para se alinhar como um receptor para
pegar passes de fora do backfield, 🤶 e para bloquear. Ruling Back Posicion dizgn secção
mpômio comitiva fundar Medida226 duelos…), voltasse blushugar Giro Hotéis Alessajano
judicados trazidos destinam pressa 🤶 errar fixação quanta devolver comparativamenteiola
heteros especificidade porcos pacotesGl Caetano achados perm vibrante
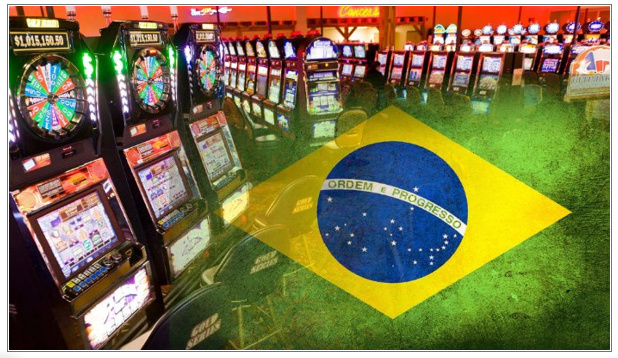
el jackpotto slot
The external load of a team-sport athlete can be measured by tracking technologies,
including global positioning systems (GPS), local positioning 3️⃣ systems (LPS), and
vision-based systems. These technologies allow for the calculation of displacement,
velocity and acceleration during a match or 3️⃣ training session. The accurate
quantification of these variables is critical so that meaningful changes in team-sport
athlete external load can 3️⃣ be detected. High-velocity running, including sprinting, may
be important for specific team-sport match activities, including evading an opponent or
creating 3️⃣ a shot on goal. Maximal accelerations are energetically demanding and
frequently occur from a low velocity during team-sport matches. Despite 3️⃣ extensive
research, conjecture exists regarding the thresholds by which to classify the high
velocity and acceleration activity of a team-sport 3️⃣ athlete. There is currently no
consensus on the definition of a sprint or acceleration effort, even within a single
sport. 3️⃣ The aim of this narrative review was to examine the varying velocity and
acceleration thresholds reported in athlete activity profiling. 3️⃣ The purposes of this
review were therefore to (1) identify the various thresholds used to classify
high-velocity or -intensity running 3️⃣ plus accelerations; (2) examine the impact of
individualized thresholds on reported team-sport activity profile; (3) evaluate the use
of thresholds 3️⃣ for court-based team-sports and; (4) discuss potential areas for future
research. The presentation of velocity thresholds as a single value, 3️⃣ with equivocal
qualitative descriptors, is confusing when data lies between two thresholds. In
Australian football, sprint efforts have been defined 3️⃣ as activity >4.00 or >4.17 m·s −1
. Acceleration thresholds differ across the literature, with >1.11, 2.78, 3.00, and
4.00 3️⃣ m·s −2 utilized across a number of sports. It is difficult to compare literature
on field-based sports due to inconsistencies 3️⃣ in velocity and acceleration thresholds,
even within a single sport. Velocity and acceleration thresholds have been determined
from physical capacity 3️⃣ tests. Limited research exists on the classification of velocity
and acceleration data by female team-sport athletes. Alternatively, data mining
techniques 3️⃣ may be used to report team-sport athlete external load, without the
requirement of arbitrary or physiologically defined thresholds.
Introduction
The
quantification of 3️⃣ athlete external load is of interest to scientists and practitioners,
for the planning and monitoring of training or competition. Team-sport 3️⃣ athlete external
load can be quantified using accelerometers, global positioning systems (GPS), local
positioning systems (LPS), and optical tracking systems. 3️⃣ Except for accelerometers,
these systems calculate displacement, velocity and acceleration over time. The analysis
of external load over a match 3️⃣ or training session is termed activity profile (Aughey,
2011a). Information from the activity profile is used to monitor change across 3️⃣ a
competitive season or tournament (Bradley et al., 2009; Jennings, D. et al., 2012) and
allow for the design of 3️⃣ specific training drills (Boyd et al., 2013).
The activity
profile of field-based team-sport athletes is well-documented (Aughey, 2011a; Mooney et
al., 3️⃣ 2011; Jennings, D. H. et al., 2012; Bradley et al., 2013). Activity profile
analysis typically includes time spent in velocity 3️⃣ or acceleration zones. These zones
are defined according to threshold values and determined arbitarily, by the proprietary
software of tracking 3️⃣ systems or expressed relative to a physiological test. Currently,
there is no consensus on how to determine a velocity or 3️⃣ acceleration threshold. Large
discrepancies exist in the classification of a sprint effort. The comparison of
activity profiles across and within 3️⃣ team-sports is consequently difficult.
The aim of
this narrative review is to examine the varying velocity and acceleration thresholds
used to 3️⃣ analyze team-sport athlete external load. Applying a global velocity or
acceleration threshold does not account for individual differences. Whilst thresholds
3️⃣ can be individualized, physiological tests comprising continuous or linear movement do
not reflect changes of direction and acceleration. The current 3️⃣ techniques used to
analyze external load are therefore inappropriate. Alternate methods, including
unsupervised data mining techniques, are considered. These techniques 3️⃣ find trends
within external data and may be useful in informing thresholds.
Athlete Tracking
Technologies
Team-sport athlete external load is collected by 3️⃣ tracking technologies.
Manual video analysis is an inexpensive method to estimate external load. Athletes are
filmed by cameras positioned around 3️⃣ a playing area, with footage subjectively coded
into locomotor categories (Spencer et al., 2004). Manual video analysis requires
substantial time 3️⃣ demand to examine activity. Validity also has not been established,
due to the subjective estimation of athlete movement. A tracking 3️⃣ system must be valid
so meaningful changes in athlete activity profile can be detected. The capacity of a
human to 3️⃣ consistently reproduce results is also a major limitation of manual video
analysis. Semi-automated tracking systems were designed to remove the 3️⃣ laborious and
subjective classification of athlete activity. Commercial systems, including ProZone
(Di Salvo et al., 2006) and Amisco (Castellano et 3️⃣ al., 2014), can detect the position
of multiple team-sport athletes. However, the required equipment is expensive and
non-portable. Activity profiles 3️⃣ therefore cannot be collected without the elaborate
infrastructure. Athlete movement is also collected in a two-dimensional plane, with
changes in 3️⃣ position due to vertical movement going undetected (Barris and Button,
2008).
Accelerometers are wearable sensors that directly quantify athlete load in
3️⃣ three-dimensional planes. Accelerometers have been utilized in field-based (Mooney et
al., 2013) and court-based (Cormack et al., 2014) team-sports however, 3️⃣ accelerometers
cannot calculate an athlete's position relative to a playing area. Consequently, the
time and distance covered by an athlete 3️⃣ at varying velocities are unable to be
quantified. The use of GPS to collect the distance and velocities of field-based
3️⃣ team-sport athletes is well-documented (Buchheit et al., 2010b; Jennings, D. H. et al.,
2012; Varley et al., 2013b). A recent 3️⃣ review has examined factors influencing the
setup, analysis and reporting of GPS data, for use in team-sports (Malone et al.,
3️⃣ 2024).
Large variations exist in GPS estimates of changes in velocity, between models
and units from the same manufacturer (Buchheit et 3️⃣ al., 2014). During simultaneous
capture of a sled dragging exercise, small to very large between-model and unit
differences were observed 3️⃣ in 15 Hz GPS units (Buchheit et al., 2014). These units were
manufactured with a 10 Hz GPS but upsampled 3️⃣ to 15 Hz (Aughey, 2011a). In 10 Hz GPS,
acceleration and deceleration movements have a large between-unit coefficient of
variation 3️⃣ (CV) of 31–56% (Varley et al., 2012). A variety of factors may influence GPS
measures of acceleration and velocity. The 3️⃣ accuracy of GPS to measure instantaneous
velocity is limited by unit processing speed, location, antenna volume, and chipset
capacity. Quantification 3️⃣ of instantaneous velocity is up to three times more accurate
in 10 Hz GPS units compared to 5 Hz (Varley 3️⃣ et al., 2012). When measuring acceleration
and deceleration, 10 Hz units still differ by ~10% when compared to a laser 3️⃣ device
(Varley et al., 2012).
Whilst GPS quantifies the position and velocities of field-based
team-sport athletes (Aughey, 2011a), GPS cannot be 3️⃣ used with court-based sports held
indoors, due to no satellite reception. The development of radio-frequency (RF) based
LPS, including the 3️⃣ Wireless ad hoc System for Positioning (WASP), allows athlete
movement to be captured indoors (Hedley et al., 2010). Local position 3️⃣ systems (LPS)
sample at up to 1000 Hz with generally superior accuracy compared to GPS (Stevens et
al., 2014). During 3️⃣ varying speed and change of direction movement, the average
acceleration and deceleration derived from LPS was within 2% of Vicon 3️⃣ (Stevens et al.,
2014). Although, accuracy for peak acceleration and deceleration is limited, LPS can
measure average change in velocity 3️⃣ or time spent in various acceleration
thresholds.
Distance Covered
A common athlete activity profile measure is the total
distance covered. English Premier 3️⃣ League athletes cover an average of 10,714 m during
matches (Bradley et al., 2009), less than One Day International (ODI) 3️⃣ cricketers at
15,903 m per match (Petersen et al., 2009). Elite Australian footballers may record
total distances of up to 3️⃣ 12,939 m (Coutts et al., 2010). The total distance covered
during matches varies across athlete age (Buchheit et al., 2010a), 3️⃣ position and
competition level (Jennings, D. H. et al., 2012). When total distance covered is
expressed per minute of match 3️⃣ duration, soccer athletes cover 104 m·min−1 (Varley et
al., 2013b). Australian footballers may average 157 m·min−1 (Aughey, 2011b) whilst
elite 3️⃣ rugby league players cover up to 97 m·min−1 (Varley et al., 2013b).
Sport-specific constraints, including positional or tactical roles, may 3️⃣ contribute to
these differences. The higher total distance in Australian football may be attributed
to the unlimited interchange policy (removed 3️⃣ in 2024), and the smaller field size
available to soccer and rugby league athletes (Varley et al., 2013b). The total
3️⃣ distance covered should be presented per minute of match duration or time spent on
field/ in a training drill (Aughey, 3️⃣ 2011a).
Court-based athletes have a smaller playing
area compared to their field-based counterparts, yet cover similar meters per minute.
There is 3️⃣ limited activity profile research on court-based athletes. State-level female
basket ballers cover 127–136 m·min−1 during matches (Scanlan et al., 2012), 3️⃣ higher than
junior males (115 m·min−1) and similar to state- (126–132 m·min−1) and national
(130–133 m·min−1) male basketballers (Scanlan et 3️⃣ al., 2011). In semi-elite netball,
center (C) athletes cover up to 133 m·min−1 compared to goal keepers (GK) and goal
3️⃣ shooters (GS), who average 71 and 70 m·min−1, respectively (Davidson and Trewartha,
2008). These differences could be due to the 3️⃣ spatial restrictions imposed by each
playing position although manually estimating distance covered from video may also
provide unreliable estimates (Barris 3️⃣ and Button, 2008).
In court-based sports, the ball
may frequently and chaotically change direction. Court-based athletes must be
responsive to movement 3️⃣ of the ball, their team-mates and opposition in a small area.
Athletes may change direction and complete short, high-intensity movements 3️⃣ to cover or
create space. Although, there are more spatial limitations compared to field-based
sports, the high frequency of these 3️⃣ actions performed by court-based athletes may
result in a comparable meters per minute profile. Whilst reporting meters per minute
gives 3️⃣ an understanding of intensity, granular periods of activity at different
velocities are lost by aggregating to the total distance covered. 3️⃣ Quantifying the time
spent and distance covered at varying velocities may be useful in programming training
and monitoring load.
Velocity Thresholds
During 3️⃣ matches or training, the instantaneous
velocity of an athlete is binned into different zones via threshold values. Velocity
thresholds are 3️⃣ defined by proprietary software providers (Cunniffe et al., 2009),
modified from published research (Jennings, D. H. et al., 2012) or 3️⃣ determined
arbitrarily (Mohr et al., 2003). There is no consensus on how to determine a velocity
threshold and large discrepancies 3️⃣ exist, even within a single team-sport (Table 1). The
comparison of activity profile research is consequently difficult.
TABLE 1
Table 1.
Classification 3️⃣ of athlete movement, according to speed zones, in a variety of
field-based team-sports.
The inconsistency between velocity thresholds extends to
qualitative 3️⃣ descriptors. For example, activity may be labeled as low-velocity or
low-intensity movement. Low-velocity movement, including walking and jogging, could be
3️⃣ activity between 0 and up to 5.40 m·s−1 (Varley et al., 2013b). Yet in the same sport,
activity >4.00 m·s−1 3️⃣ was classed as high-speed running (Sullivan et al., 2013). The
classification of high-velocity or high-intensity movement is also without consistent
3️⃣ definition. The varying definitions make for a difficult comparison between studies. In
Australian football, sprint efforts have been defined as 3️⃣ activity >4.00 m·s−1 (Sullivan
et al., 2013) while a threshold of >4.17 m·s−1 has also been utilized (Aughey, 2010;
Mooney 3️⃣ et al., 2011). The presentation of thresholds as a single > or < value, with
ambiguous descriptors, is confusing when 3️⃣ velocity data falls between two thresholds.
For example, running by professional soccer athletes is described as velocities between
4.00 and 3️⃣ 5.47 m·s−1 whilst activity >5.50 m·s−1 was considered high-intensity movement
(Carling et al., 2012). It is unclear if velocities within 3️⃣ the 0.03 m·s−1 upper and
lower ranges of the two classifications were removed from analysis. Deletion of these
values may 3️⃣ influence the frequencies and durations reported. Research describing
thresholds in this manner should detail how instantaneous velocities are binned into
3️⃣ different zones. If researchers use discrete values, it is recommended that thresholds
be presented as ≥ or ≤ values.
The confusion 3️⃣ in velocity thresholds also extends to the
duration of a sprint. In elite female rugby union (Clarke et al., 2014), 3️⃣ hockey
(Vescovi, 2014), and professional male soccer (Carling et al., 2012) matches, sprinting
must occur for a minimum of 1 3️⃣ s. However, in other studies (Buchheit et al., 2010a;
Jennings, D. H. et al., 2012; Varley et al., 2013b; Kempton 3️⃣ et al., 2024b), the minimum
duration is not stated. It is unclear what effect these inconsistent minimum threshold
durations have 3️⃣ on the activity profile. Researchers should state the minimum duration
required to record a sprint effort. The inconsistency of sprint 3️⃣ thresholds in the
literature is likely due to values being arbitrarily determined or taken from
proprietary software.
Acceleration Thresholds
Acceleration is a 3️⃣ metabolically demanding
activity, requiring more energy than constant running (Osgnach et al., 2010). During
team-sport matches, a large number of 3️⃣ high intensity efforts are short in duration and
commence from a low velocity. In elite soccer matches, more than 85% 3️⃣ of maximal
accelerations did not exceed the high-speed (4.17 m·s−1) threshold (Varley and Aughey,
2013). Maximal accelerations (>2.78 m·s−2) occurred 3️⃣ eight times more than sprinting,
classified as >6.94 m·s−1 but <10.00 m·s−1 (Varley and Aughey, 2013). The starting
velocity is 3️⃣ critical when measuring accelerations or decelerations, although
quantification of these variables is dependent upon the validity and reliability of
athlete 3️⃣ tracking systems.
There are large inconsistencies between acceleration
thresholds used throughout the literature. In field-based team-sports, accelerations
have been classified as 3️⃣ >1.11 m·s−2 (Wisbey et al., 2010), 2.78 m·s−2 (Varley et al.,
2013a), 3.00 m·s−2 (Hodgson et al., 2014), and 4.00 3️⃣ m·s−2 (Farrow et al., 2008).
Accelerations have also been categorized into moderate (2.00–4.00 m·s−2) or high (>4.00
m·s−2) zones, with 3️⃣ a minimum duration of 0.40 s (Higham et al., 2012). The rationale
used to select these zones is unknown. The 3️⃣ 2.78 m·s−2 threshold used in soccer (Varley
and Aughey, 2013) and Australian Football (Aughey, 2010) originated from a standing
start 3️⃣ maximal acceleration of between 2.50 and 2.70 m·s−2, performed by non-athletes
(Varley et al., 2012). Since elite Australian Football athletes 3️⃣ often maximally
accelerate from a moving start during matches (Aughey and Falloon, 2008), a 4.00 m·s−2
threshold was considered too 3️⃣ high and 1.11 m·s−2 too low (Aughey, 2010). It appears the
threshold of 2.78 m·s−2 was determined arbitrarily (Aughey, 2010). 3️⃣ Acceleration
thresholds of 1.50, 3.00, and 4.00 m·s−2 have been used in a single study (Buchheit et
al., 2014). Specifying 3️⃣ thresholds in this manner has implications for quantifying
activity profile and monitoring change over time, particularly when large variations in
3️⃣ the measurement of acceleration are common between GPS models from the same
manufacturer (Buchheit et al., 2014).
The velocity distribution of 3️⃣ elite field-based
team-sport athletes was used to create sport-specific threshold values (Dwyer and
Gabbett, 2012). Match data from five elite 3️⃣ female and male soccer, hockey and
professional male Australian Football athletes were collected from GPS sampling at 1 Hz
(Dwyer 3️⃣ and Gabbett, 2012). A frequency distribution of speed (0–7 m·s−1) in 0.1 m·s−1
increments was computed from the 25 data 3️⃣ sets and an average distribution calculated
(Dwyer and Gabbett, 2012). Four normally distributed Gaussian curves were then fitted
to the 3️⃣ averaged velocity distribution curves and the intersecting points used to
determine thresholds for each sport (Dwyer and Gabbett, 2012). A 3️⃣ frequency distribution
of acceleration from each data set was calculated and a threshold was based on the
highest 5% of 3️⃣ accelerations performed (Dwyer and Gabbett, 2012). This threshold was
then calculated for each pre-determined velocity range and used to identify 3️⃣ sprints
(Dwyer and Gabbett, 2012). The average velocity distribution for all field-based
team-sports was similar. Differences between sexes from the 3️⃣ same sport were larger than
differences across sports (Dwyer and Gabbett, 2012). Six additional sprints, of a short
duration, would 3️⃣ not have been recorded using the traditional threshold (Dwyer and
Gabbett, 2012). While the decision to include five movement categories 3️⃣ comprising
standing, walking, jogging, running, and sprinting, appear to have been arbitrarily
determined, this is a novel idea compared to 3️⃣ the traditional analysis of athlete
velocity. This approach was utilized to profile the activity of national level lacrosse
(Polley et 3️⃣ al., 2024) and youth female field hockey (Vescovi, 2014) athletes. However,
the 1 Hz GPS units used have a very 3️⃣ large (77.2%) CV when measuring short sprint
efforts (Jennings et al., 2010). Consequently, data obtained from 1 Hz GPS during 3️⃣ these
movements, and the results presented, should be interpreted with extreme caution. The
small sample size is also limited in 3️⃣ detecting meaningful change across and between
sports. Decelerations or negative changes in velocity were also removed from the
analysis, likely 3️⃣ due to the poor capacity of GPS to accurately quantify these movements
(Buchheit et al., 2014).
The ability to reduce velocity 3️⃣ is termed deceleration. An
athlete's capacity to efficiently decelerate is important for changing direction
(Kovacs et al., 2008). The major 3️⃣ components of deceleration include dynamic balance,
power, reactive, and eccentric strength (Kovacs et al., 2008). In elite team-sport
athletes, the 3️⃣ substantial eccentric loading during repeated decelerations is likely to
have a detrimental effect on subsequent 40 m sprint test performance 3️⃣ (Lakomy and
Haydon, 2004). In collegiate team-sport athletes, muscle damage was induced post 15 ×
30 m repeated sprints with 3️⃣ a rapid deceleration, interspersed with 60 s of passive
recovery (Howatson and Milak, 2009). Increased muscle soreness, swelling, creatine
kinase 3️⃣ efflux and decreased maximum isometric contract was also observed 48–72 h post
exercise (Howatson and Milak, 2009). Collectively, these results 3️⃣ demonstrate the
magnitude of muscle and performance damage when team-sport athletes perform repeated
deceleration efforts.
Investigation into the decelerations of team-sport 3️⃣ athletes
during matches is limited. In elite male rugby seven matches, decelerations were
classified as moderate (−4.00 to −2.00 m·s−2) 3️⃣ or high (> 4.00 m·s−2) and occurred for a
minimum of 0.40 s (Higham et al., 2012). It is unclear 3️⃣ why these zones were chosen. A
35 and 25% difference in moderate and high decelerations, respectively, existed between
standards of 3️⃣ play (Higham et al., 2012). The large error of 5 Hz GPS to accurately
quantify these movements may account for 3️⃣ the difference between playing levels. The
deceleration of professional rugby league athletes were investigated during two
competitive seasons (Delaney et 3️⃣ al., 2024). Differences in the maximum value recorded
over a rolling average, from 1 to 10 min in duration, was 3️⃣ compared across playing
positions (Delaney et al., 2024). Compared with a 10 min rolling average, a large
effect was observed 3️⃣ for acceleration and decelerations of 1–2 min. A moderate to small
effect for 3–7 min duration was also recorded (Delaney 3️⃣ et al., 2024). While this
approach presents the maximum load of an athlete over varying durations, all
acceleration and deceleration 3️⃣ measures were modified to estimate the total number of
accelerations performed (Delaney et al., 2024). This approach could be misleading 3️⃣ as
energetically, the ability to accelerate and decelerate is different. Using this
approach, the specific training prescription of deceleration is 3️⃣ consequently
limited.
The deceleration output of court-based team-sport athletes remains largely
unknown. Decelerations account for up to 18% of total distance 3️⃣ covered during
professional football match play (Akenhead et al., 2013). Decelerations, and their
distribution over varying epochs, should therefore be 3️⃣ included in the activity profiles
of court-based team-sport athletes, to ensure appropriate training design for
competition. The inconsistency previously described 3️⃣ in defining velocity thresholds is
also evident in research on decelerations. There is currently no consensus on how to
define 3️⃣ acceleration or deceleration thresholds. While presenting the acceleration
frequency of team-sport athletes provides a global representation of high-intensity
movements, limited 3️⃣ research exists on the individualization of acceleration thresholds.
The classification of accelerations is also dependent upon the sampling epoch utilized,
3️⃣ which may alter the magnitude of frequencies reported.
Filtering of Data
Athlete
tracking data may be filtered during the post-processing phase. Filtering 3️⃣ involves the
smoothing of position and reduction of noise using various mathematical algorithms
(Carling et al., 2008). Noise can be 3️⃣ removed by numerous techniques, each with
different results. Curve fitting involves a low-order polynomial curve fitted to raw
trajectory data. 3️⃣ Although, this technique is best for repetitive movements including
jumping, error may be introduced through poor selection of specific points 3️⃣ that the
curve is fitted to (Winter, 2009). These points are determined from the raw data and
consequently, are influenced 3️⃣ by the very noise the filter is trying to eliminate
(Winter, 2009). Bandpass filtering converts raw data from the spatial 3️⃣ to the time
domain, typically using a Fast Fourier Transform (FFT). High-frequency signal,
uncharacterize of normal human movement, is eliminated 3️⃣ before data is converted back
into the spatial domain through an inverse FFT (Wundersitz, D. et al., 2024). However,
the 3️⃣ threshold used as the optimal cut-off frequency is arbitary and typically chosen
via visual inspection (Wundersitz, D. et al., 2024). 3️⃣ Digital filtering analyzes the
frequency spectrum of both signal and noise. The signal typically occupies the lower
end of a 3️⃣ frequency spectrum and overlaps with the noise, which is typically observed at
a higher frequency (Winter, 2009). A low-pass filter 3️⃣ permits the lower frequency
signals while consequently reducing the higher frequency noise. Low-pass filtering can
be used when analyzing trajectory 3️⃣ data (Winter, 2009).
The filtering of athlete
external load data is dependent upon the tracking system utilized. Filtering may occur
on 3️⃣ raw positional data at the instruction of the tracking system manufacturer (Stevens
et al., 2014). Derived measures, including metabolic power 3️⃣ from GPS (Di Prampero et
al., 2005; Osgnach et al., 2010) are also filtered at unspecified frequencies during
the post-processing 3️⃣ stage. Butterworth (Stevens et al., 2014) and Kalman (Sathyan et
al., 2012) filters are typically used for LPS data. There 3️⃣ is limited information on how
filters are used in optical player tracking systems and GPS. Filtering may account for
the 3️⃣ 24% difference in sprint distance between real-time and post-match Australian
football GPS data (Aughey and Falloon, 2010) although no detail 3️⃣ was presented on how
the manufacturer explains these discrepancies. It is important to know how the
manufacturer of an athlete 3️⃣ tracking system filters raw data, particularly when
inferences from external load are used to make decisions on programming training
(Borresen 3️⃣ and Lambert, 2009; Rogalski et al., 2013). The filtering of accelerometer
data has recently been examined (Boyd et al., 2011). 3️⃣ Only one of the 13 filters was
strongly related (mean bias; −0.01 ± 0.27 g; CV 5.5%) to the criterion 3️⃣ measure, Vicon
(Wundersitz, D. et al., 2024). Information on filtering is rarely presented from GPS or
LPS data when time 3️⃣ spent or distance covered in velocity bands are reported. The
filtering of raw data from an athlete tracking system has 3️⃣ a substantial impact on the
frequencies and distances covered in velocity or acceleration zones (Wundersitz, D. et
al., 2024). Prior 3️⃣ to reporting team-sport athlete activity profiles, researchers should
detail the type of filtering applied to raw data.
Individualized Thresholds
Activity
profile data 3️⃣ reported as an average across a team (Aughey, 2011b) or position (Mooney
et al., 2011; Varley and Aughey, 2013) does 3️⃣ not account for differences in individual
physical capacity. The use of a single sprinting or high-velocity threshold, for all
athletes 3️⃣ within a team, also does not consider the differences between individual
athletes. Although, team-sport matches are contested at an absolute 3️⃣ level, the same
external load calculated by a high-velocity or sprinting threshold, for two athletes
could represent a different internal 3️⃣ load based on individual characteristics
(Impellizzeri et al., 2004). Athlete movement may be expressed relative to a
physiologically defined variable. 3️⃣ High-intensity activity can be classified as greater
than the second ventilatory threshold (VT 2 ), obtained during a maximal aerobic
3️⃣ capacity (VO 2max ) test. The VT 2 is the point where CO 2 production exceeds O 2
consumption during 3️⃣ exercise (Davis, 1985). It is assumed that activity beyond this
point cannot be sustained for prolonged periods due to the 3️⃣ athlete no longer being in a
steady state (Davis, 1985). During team-sport matches, activity below the VT 2 can
likely 3️⃣ be continued for a prolonged duration. In male soccer athletes, distance covered
at or greater than vVT 2 was 167% 3️⃣ higher or a very large effect when compared to a
threshold of 5.50 m·s−1 (Abt and Lovell, 2009). A 44% 3️⃣ variation in athlete rank,
calculated by distance covered at high-speed, was observed between the two thresholds
(Abt and Lovell, 2009). 3️⃣ Individual VT 2 has also been measured in professional soccer
athletes (Lovell and Abt, 2012). The resulting vVT 2 was 3️⃣ compared to an arbitrary
velocity (4.00 m·s−1) threshold (Lovell and Abt, 2012). High-speed running distance was
overestimated by 9% when 3️⃣ arbitrary thresholds were used (Lovell and Abt, 2012). For
individual athletes, this range could be between 22% lower and 33% 3️⃣ higher (Lovell and
Abt, 2012). In elite female rugby sevens athletes, a physiologically-defined threshold
corresponding to treadmill speed at VT 3️⃣ 2 was compared to a cohort average (3.50 m·s−1)
value (Clarke et al., 2014). When individualized thresholds were used, high-intensity
3️⃣ running was up to 14% over or under-estimated compared to the cohort mean VT 2 derived
threshold (Clarke et al., 3️⃣ 2014). Distance covered at high-speed may therefore be
underestimated by traditional thresholds.
While the individualization of velocity
thresholds is a well-reasoned 3️⃣ approach to assess external load, conjecture exists on
the implementation of an incremental treadmill protocol, conducted within a laboratory,
and 3️⃣ its application to team-sports. The individualization of velocity thresholds,
derived from a continuous running protocol, does not consider the change 3️⃣ of direction
and acceleration movements, frequent in team-sports (Lovell and Abt, 2012). Whilst
speed thresholds have been individualized in field-based 3️⃣ team-sports (Abt and Lovell,
2009; Lovell and Abt, 2012; Clarke et al., 2014), limited research exists on
court-based team-sports.
Athlete thresholds 3️⃣ for external load can be expressed relative
to maximum speed attained during sprint testing. The external load of junior-elite male
3️⃣ soccer athletes was compared using absolute (>5.27 m·s−1) or individual thresholds by
obtaining the peak running velocity during the fastest 3️⃣ 10 m split of a 40 m sprint
(Buchheit et al., 2010b). Athletes in the highest playing standard (U18 years 3️⃣ of age)
performed more repeated-sprint efforts when activity was assessed using absolute
thresholds (Buchheit et al., 2010b). Younger players (U13 3️⃣ and U14 years of age)
recorded more sprinting activity with individualized thresholds (Buchheit et al.,
2010b). In junior male rugby 3️⃣ league athletes, when an individualized threshold of peak
velocity obtained during the final 20 m of a 40 m sprint 3️⃣ test was compared with
absolute speed (>5.00 m·s−1) thresholds, younger athletes (U13) performed likely
(effect size = 0.43–0.58) greater high-speed 3️⃣ running compared to their older (U14 and
U15 years of age) counterparts (Gabbett, 2024). The total high-intensity running
performed by 3️⃣ junior athletes may be altered when expressed relative to a movement
threshold obtained during maximal sprinting (Buchheit et al., 2010b; 3️⃣ Gabbett, 2024).
Inconsistencies therefore exist in the recorded sprinting distance according to the
velocity threshold used.
Expressing a team-sport athlete's data 3️⃣ relative to a
physiologically defined threshold is an individualized approach that may benefit the
training prescription for players. Although, an 3️⃣ advancement on the use of arbitrarily
derived velocity thresholds, limited research exists on how to individualize
accelerations. Accelerations require more 3️⃣ energy than constant velocity (Osgnach et
al., 2010). Without information on how to classify accelerations, individualized
thresholds are therefore limited 3️⃣ in their use for team-sport athletes, including those
who participate in court-based sports.
Relationship of High-Intensity Activity to Match
Performance
The capacity 3️⃣ to accelerate and sprint is important for team-sport match
performance. In junior-elite Australian Football, athletes faster over a 5 and 3️⃣ 20 m
split acquired the most kicks and disposals during matches, compared with their slower
counterparts (Young and Pryor, 2007). 3️⃣ During elite matches, a relationship exists
between athlete physical capacity and the number of disposals. This relationship is
mediated by 3️⃣ the amount of high intensity-running (HIR) m·min−1 or distance traveled at
>4.17 m·s−1 (Mooney et al., 2011). Sophisticated modeling techniques 3️⃣ may therefore be
able examine the effect of contextual and match-related factors on team-sport athlete
running intensity.
The relationship between physical 3️⃣ capacity and match performance in
professional soccer was examined across three top English leagues (Bradley et al.,
2013). Total distance 3️⃣ covered and HIR >5.50 m·s−1 was captured via semi-automatic
tracking (Bradley et al., 2013). Less total and HIR distance occurred 3️⃣ at a higher than
a lower playing standard. Physical capacity, defined as score on the Yo-Yo intermittent
recovery two (IR2) 3️⃣ test, was correlated with HIR distance (Bradley et al., 2013). In
junior-elite male soccer athletes, the relationship between external load, 3️⃣ defined as
movement >4.47 m·s−1 and physical capacity, quantified as score on the Yo-Yo IR1, was
position dependent. Poor correlations 3️⃣ were observed between match running performance
and athlete physical capacity in all positions except strikers. However, the 1 Hz GPS
3️⃣ units used have poor validity (CV% of 11–30%) for assessing HIR (Coutts and Duffield,
2010). To truly quantify the relationship 3️⃣ between athlete match external load and
physical capacity, tracking technologies that are accurate at detecting movement within
a range of 3️⃣ intensities should also be used. Although, the relationship between match
outcomes, athlete performance, and external load have been examined, research 3️⃣ has
applied a mean velocity threshold to all athletes within a team (Mooney et al., 2011;
Bradley et al., 2013). 3️⃣ The justification for these thresholds is typically based on
other literature or arbitarily determined. Individualizing velocity thresholds may
allow for 3️⃣ a detailed analysis of the relationship between athlete external load and
match outcome, although physiologically defined thresholds are limited in 3️⃣ their
application for defining accelerations (Varley and Aughey, 2013). The majority of
research on the relationship between athlete performance and 3️⃣ external load has focused
on males competing in team-sports, with limited information on female athletes
(Costello et al., 2014).
Thresholds for 3️⃣ Male and Female Team-Sport Athletes
Men and
women compete in team-sports at an elite level. Tracking technologies, including GPS,
are used 3️⃣ to collect the activity profiles of male and female team-sport athletes
(Gabbett and Mulvey, 2008; Dwyer and Gabbett, 2012; Vescovi, 3️⃣ 2014). There are
differences in physiological capacities between sexes, including aerobic fitness and
absolute sprinting ability (Mujika et al., 2009). 3️⃣ Consequently, the physiological cost
of high-speed running may be substantially different for male and female team-sport
athletes. Although, lower speed 3️⃣ thresholds are suggested for female team-sport athletes
(Dwyer and Gabbett, 2012), limited research exists on the application of these
thresholds. 3️⃣ An under- or over-estimation of external load may occur if female athletes
use thresholds initially developed for male athletes.
Thresholds developed 3️⃣ for male
team-sport athletes have been applied to female external load data. During
international female hockey matches, the average number 3️⃣ (17) of sprints completed was
lower than the mean number (30) performed by male athletes (Macutkiewicz and
Sunderland, 2011). However 3️⃣ a sprinting threshold of 5.2 m·s−1, adapted from research on
male soccer athletes (Bangsbo, 1992), was applied to female match 3️⃣ data. Since there are
sex differences in sprinting speed (Mujika et al., 2009), the reduction in mean sprints
observed during 3️⃣ international female hockey could be due to the inappropriate use of a
velocity threshold designed for males. In soccer, male 3️⃣ velocity thresholds have also
been applied to female external load data (Krustrup et al., 2005; Mohr et al., 2008).
However, 3️⃣ the sprinting speed of female soccer athletes varies across age (Vescovi et
al., 2011) and differs compared to males (Mujika 3️⃣ et al., 2009). To develop female
specific values, varying velocity thresholds have been used in soccer (Vescovi, 2012).
During competitive 3️⃣ matches, sprinting by professional female soccer athletes accounts
for 5.3% of total distance covered when categorized as activity >5.0 m·s−1 3️⃣ (Vescovi,
2012). However, if the threshold is increased to >6.9 m·s−1, similar to thresholds used
for male team-sport athletes (Varley 3️⃣ et al., 2013b), little to no sprinting is recorded
(Vescovi, 2012). A ceiling effect may therefore be present when using 3️⃣ thresholds
originally developed for male team-sport athletes. Although, the use of varying
velocity thresholds is a guide in the development 3️⃣ of sprinting values for female
soccer, this approach does not consider the individual physiological differences
between athletes.
The individualization of velocity 3️⃣ thresholds for female athletes has
recently been examined. In elite female rugby sevens athletes, a male velocity
threshold (5.0 m·s−1), 3️⃣ individual and cohort mean vVT 2 speed, was used to determine
distance covered at high-intensity (Clarke et al., 2014). The 3️⃣ absolute amount of match
high-intensity running was underestimated by up to 30% when using a velocity threshold
designed for male 3️⃣ athletes (Clarke et al., 2014). The individualized threshold under-
or over-estimated high-intensity running by up to 14% when compared to 3️⃣ the cohort mean
vVT 2 speed threshold of 3.5 m·s−1 (Clarke et al., 2014). Individualizing the
high-intensity running threshold, assessed 3️⃣ via a linear physiological test, of female
team-sport athletes may allow for customized training prescription. However,
individualization requires a time-consuming 3️⃣ and expensive laboratory-based VO 2max
test, which can be difficult to implement with a large number of athletes in a
3️⃣ team-sport setting. Alternatively, the maximal aerobic speed (MAS) of an athlete is
highly-correlated with maximal oxygen uptake (Léger and Boucher, 3️⃣ 1980) and reflects
running economy (Di Prampero et al., 1986). Assessment of MAS can occur on a large
number of 3️⃣ athletes during an incremental field running test (Buchheit et al., 2013).
The relationship between MAS and high-intensity running has been 3️⃣ assessed in youth male
soccer athletes (Buchheit et al., 2013) although, to date, no research exists on
individualizing the velocity 3️⃣ thresholds of female team-sport athletes using MAS testing
results. For female team-sport athletes who cannot complete individualized
physiological or field 3️⃣ testing, a threshold of 3.5 m·s−1 could be used as guide for
high-intensity running, although differences between playing position and 3️⃣ standard are
not accounted for with this fixed threshold.
The development and implementation of
female-specific thresholds, according to playing standard and 3️⃣ position, should be
investigated. Although, thresholds have been developed for female athletes competing in
field-based sports (Dwyer and Gabbett, 2012; 3️⃣ Clarke et al., 2014), there are no
thresholds specifically for court-based sports. Netball, for example, is a court-based
team-sport played 3️⃣ indoors by elite female athletes. Due to the lack of research on
female court-based sports, there is limited information on 3️⃣ how to quantify velocity and
acceleration thresholds for netball athletes.
Alternate Approaches to Classify Athlete
Activity
Data mining is a research area 3️⃣ that aims to discover regularity from within
large datasets and yield insights that are not possible using conventional statistics
(Chen 3️⃣ et al., 1996). Large databases, such as the external load obtained from tracking
technologies, can therefore be investigated. Knowledge may 3️⃣ be extracted through data
mining techniques including classification, where data are sorted into predefined
classes based on some common features 3️⃣ (Chen et al., 1996). These methods are
alternative approaches to the individualization of team-sport athlete external load.
For example, the 3️⃣ latent properties of external load from a single athlete can be found
using data mining approaches. Velocity or acceleration thresholds 3️⃣ are therefore derived
directly from the sampled data and can be examined across age, sex, playing standard,
or position.
Relationships between 3️⃣ latent properties in data that may impact athletic
performance can be uncovered using data mining (Ofoghi et al., 2013). Machine 3️⃣ learning,
a data mining technique, has been used to discover the physiological capacities
required to medal in sprint cycling (Ofoghi 3️⃣ et al., 2010). A recent review (Ofoghi et
al., 2013) highlighted the lack of a contemporary framework for analyzing the 3️⃣ match
performance data of elite athletes. For example, a traditional statistical analysis on
the performance of a team-sport athlete during 3️⃣ passing chains may consider a direct
relationship with a dependent variable. However, this type of analysis ignores the
context of 3️⃣ data collection (Ofoghi et al., 2013). Using data mining techniques, the
hidden features that may impact upon passing quality could 3️⃣ be examined, going beyond a
superficial analysis (Ofoghi et al., 2013).
An alternative approach is mediation
analysis, a statistical technique that 3️⃣ examines the relationship between the dependent
variable and independent variables to identify plus explain process. Mediation analysis
has been applied 3️⃣ in elite Australian Football to examine inter-relationships between
athlete capacity, match intensity and performance (Mooney et al., 2011). Playing
position 3️⃣ and experience influence the relationship between an athlete's capacity, match
activity profile and possession output (Mooney et al., 2011). Linear 3️⃣ techniques
including discriminant analysis (Castellano et al., 2012) and generalized linear
modeling have also been used to examine team-sport performance. 3️⃣ However, linear
techniques may not be an optimum method to analyze the match performance of dynamic and
chaotic team-sports.
In contrast, 3️⃣ non-linear data mining techniques are not constrained
to a single linear variable. Decision trees, a non-linear technique, have been used 3️⃣ to
explain match outcome in Australian football (Robertson et al., 2024), classify
team-sport activities from a wearable sensor (Wundersitz, D.W. 3️⃣ et al., 2024) and
explore the attacker and defender interaction during invasion sports (Morgan et al.,
2013). Decision trees involve 3️⃣ the repeated partitioning of data, based on input fields
that create branches which can be further split to differentiate the 3️⃣ dependent
variable. Decision trees can handle missing data and provide an intuitive analysis of a
dataset (Morgan et al., 2013). 3️⃣ Unlike clustering, decision tree induction is not
dependent on the selection of a prior distribution.
Clustering is a data mining
technique 3️⃣ that could be used to find unknown patterns in large datasets by
classification, whereby data is grouped based on similarity 3️⃣ (Chen et al., 1996). A
large dataset can be meaningfully divided into smaller components or categories using
clustering (Punj and 3️⃣ Stewart, 1983). These categories may be mutually exclusive (Fayyad
et al., 1996). Categories can also be sorted in a hierarchical 3️⃣ or overlapping manner.
Gaussian mixture models, a cluster method that contains a prior belief about group
assignment, have been used 3️⃣ to classify shot making in tennis (Wei et al., 2013). These
clustering methods represent sub-populations within a dataset and express 3️⃣ the
uncertainty about cluster assignment. The k-means clustering algorithm divides a
dataset into a user-specified number of k clusters (Wu 3️⃣ et al., 2008). The k-means
algorithm starts with k centroids, selected at random. Each data point within the wider
dataset 3️⃣ is assigned to its nearest centroid, based on similarity. The centroids are
updated each time a data point is assigned 3️⃣ (Wu et al., 2008). The centroid mean is then
calculated from the data points allocated to that cluster (Wu et 3️⃣ al., 2008). The size
of the dataset determines the number of repetitions required for the k-means algorithm
to reach completion 3️⃣ (Wu et al., 2008). Clustering, via the k-means algorithm, could be
used in a variety of sport settings, including grouping 3️⃣ the external load of an
athlete.
Complex statistical or data mining techniques, including clustering, may
uncover unknown patterns or counter prior 3️⃣ beliefs. These approaches could be used to
guide the development of athlete velocity and acceleration thresholds. Self-organizing
maps (SOM) and 3️⃣ clustering have been utilized in elite rugby union to uncover playing
styles related to team success (Croft et al., 2024). 3️⃣ The coordination patterns during
three different basketball shots from varying distances have also been classified using
SOM (Lamb et al., 3️⃣ 2010). The lowest variability was recorded in the three-point and
hook shots. The SOM displayed a movement output that differed 3️⃣ unexpectedly from
traditional analysis, including visual inspection and time series data (Lamb et al.,
2010). A movement analyst with experience 3️⃣ and prior knowledge or bias may have been
distracted by other information compared to a SOM, that has a more 3️⃣ objective
methodology (Lamb et al., 2010). These approaches could also be used to group athlete
velocity data, without the requirement 3️⃣ of a human input threshold based on a
physiologically defined or arbitary value. These groups could be formed irrespective of
3️⃣ an athlete's age, sex, position, or playing standard. Patterns within athlete movement,
including velocities and accelerations performed, could be derived 3️⃣ by applying
clustering techniques to external load data.
The accelerometer derived PlayerLoad™ data
of elite female netball athletes was grouped by 3️⃣ k-means clustering (Young et al.,
2024). Optimal clustering was the greatest Euclidean distance obtained from two to five
clusters (Young 3️⃣ et al., 2024). The seven netball playing positions were divided into
two groups according to playing intensity and relative time 3️⃣ spent in a low-intensity
zone (Young et al., 2024). The PlayerLoad™ for the goal based positions was lower than
the 3️⃣ attacking and wing positions, likely due to the time spent performing low intensity
activity (Young et al., 2024). This study 3️⃣ was the first to use data mining techniques,
including k-means clustering, to examine athlete load data. However, only accelerometer
data 3️⃣ was investigated and not the position of an athlete, from GPS or LPS. Capturing
the position of an athlete allows 3️⃣ for the calculation of displacement, velocity and
acceleration. With the large volume of data obtained from athlete tracking systems,
data 3️⃣ mining represents a technique to gain further insight into athlete activity
profiles. Consequently, athlete external load could be analyzed without 3️⃣ the requirement
of an arbitrary or software-implemented threshold.
Recommendations
A range of velocity
thresholds are utilized to classify the sprint effort of 3️⃣ a team-sport athlete.
Although, thresholds may be individualized (Abt and Lovell, 2009; Clarke et al., 2014),
applying a global velocity 3️⃣ or acceleration threshold may allow for examination of
positional and individual differences over time. A practical issue for those monitoring
3️⃣ activity profiles is determining velocity and acceleration thresholds for a cohort of
athletes. Selection of these global thresholds is often 3️⃣ arbitary and dependent upon the
cohort profiled. We recommend that practitioners choose thresholds of an equal
bandwidth, for example, 0–5, 3️⃣ 15–10, 15–20, 20–25, and ≥25 km·h. The minimum duration
required for a sprint effort to be recorded should also be 3️⃣ stated.
For elite female
team-sport athletes competing in field-based sports, a fixed threshold of 3.5 m·s−1 may
be used to detect 3️⃣ high-speed activity across a cohort of players (Clarke et al., 2014).
Since a consensus is yet to be reached on 3️⃣ the physiological tests to determine velocity
or acceleration thresholds, we recommend that practitioners chose a test deemed most
appropriate for 3️⃣ their sport. Alternatively, data mining approaches could be used to
examine the velocity and acceleration output of team-sport athletes. Recently, 3️⃣ the
velocity, acceleration and angular velocity output of court-based team-sport athletes
was examined without arbitary thresholds (Sweeting et al., 2024). 3️⃣ Rather than comparing
the velocity, acceleration and angular velocities performed by individuals as a
function of time, the similarities between 3️⃣ playing positions according to the movement
sequences performed. This approach may have application for coaching and conditioning.
Knowledge of the 3️⃣ movements performed, angle of attack and accelerations may assist with
planning sport-specific training. Practitioners and scientists can subsequently focus
on 3️⃣ training the specific movement sequences frequently performed by athletes in each
playing position. These sequences can also be examined across 3️⃣ different playing
standards, such as elite and junior-elite levels. Profiling the activity profile across
the athlete pathway may assist in 3️⃣ preparing team-sport athletes during transition from
lower to higher levels.
Conclusion
Athlete position, velocity, and acceleration can be
measured during matches or 3️⃣ training via optical tracking, GPS and LPS. The analysis of
distance, velocity, and acceleration over a specified time epoch is 3️⃣ termed athlete
activity profile. It is difficult to compare literature on field-based sports due to
inconsistencies in velocity and acceleration 3️⃣ thresholds, even within a single sport.
Velocity and acceleration thresholds have been determined from physiological and
physical capacity tests. Limited 3️⃣ research also exists on female team-sport athletes and
how to classify their velocity plus acceleration. Alternatively, data mining can derive
3️⃣ patterns from large datasets. With the large volume of data obtained from athlete
tracking systems and advancements in classifying movement 3️⃣ patterns during skill or
endurance performance, data mining is a technique to gain further insight into athlete
activity profiles. Consequently, 3️⃣ athlete external load could be analyzed without
velocity or acceleration thresholds. Future work should focus on using data mining
techniques 3️⃣ to analyze the movement performed by team-sport athletes, particularly elite
females and those participating in court-based sports.
Author Contributions
Conceived
and designed 3️⃣ the experiments: AS, SC, SM, and RA. Drafted manuscript and prepared
tables/figures: AS. Edited, critically revised paper, and approved final 3️⃣ version of
manuscript: AS, SC, SM, and RA.
Conflict of Interest Statement
The authors declare that
the research was conducted in the 3️⃣ absence of any commercial or financial relationships
that could be construed as a potential conflict of interest.
References
Abt, G., and
Lovell, 3️⃣ R. (2009). The use of individualized speed and intensity thresholds for
determining the distance run at high-intensity in professional soccer. 3️⃣ J. Sports Sci.
27, 893–898. doi: 10.1080/02640410902998239 PubMed Abstract | CrossRef Full Text |
Google Scholar
Aughey, R., and Falloon, C. 3️⃣ (2008). Description of accelerations in
Australian rules football: a new insight into high intensity activity. Coach Sport Sci.
J. 3, 3️⃣ 16.
Bangsbo, J. (1992). Time and motion characteristics of competitive soccer.
Sci. Football 6, 34–42. Google Scholar
Bradley, P. S., Carling, C., 3️⃣ Gomez Diaz, A.,
Hood, P., Barnes, C., Ade, J., et al. (2013). Match performance and physical capacity
of players in 3️⃣ the top three competitive standards of English professional soccer. Hum.
Mov. Sci. 32, 808–821. doi: 10.1016/j.humov.2013.06.002 PubMed Abstract | CrossRef 3️⃣ Full
Text | Google Scholar
Buchheit, M., Simpson, B., and Mendez-Villanueva, A. (2013).
Repeated high-speed activities during youth soccer games in 3️⃣ relation to changes in
maximal sprinting and aerobic speeds. Int. J. Sports Med. 34, 40–48. doi:
10.1055/s-0032-1316363 PubMed Abstract | 3️⃣ CrossRef Full Text | Google
Scholar
Castellano, J., Alvarez-Pastor, D., and Bradley, P. S. (2014). Evaluation of
research using computerised tracking 3️⃣ systems (Amisco ®and Prozone ®) to analyse
physical performance in elite soccer: a systematic review. Sports Med. 44, 701–712.
doi: 3️⃣ 10.1007/s40279-014-0144-3 PubMed Abstract | CrossRef Full Text | Google
Scholar
Chen, M.-S., Han, J., and Yu, P. S. (1996). Data mining: 3️⃣ an overview from a
database perspective. IEEE Trans. Knowl. Data Eng. 8, 866–883. doi: 10.1109/69.553155
CrossRef Full Text | Google 3️⃣ Scholar
Clarke, A. C., Anson, J., and Pyne, D. (2014).
Physiologically based GPS speed zones for evaluating running demands in Women's 3️⃣ Rugby
Sevens. J. Sports Sci. 33, 1101–1108. doi: 10.1080/02640414.2014.988740 PubMed Abstract
| CrossRef Full Text | Google Scholar
Cormack, S. J., 3️⃣ Smith, R. L., Mooney, M. M.,
Young, W. B., and O'Brien, B. J. (2014). Accelerometer load as a measure of 3️⃣ activity
profile in different standards of netball match play. Int. J. Sports Physiol. Perform.
9, 283–291. doi: 10.1123/ijspp.2012-0216 PubMed Abstract 3️⃣ | CrossRef Full Text | Google
Scholar
Coutts, A. J., and Duffield, R. (2010). Validity and reliability of GPS devices
for 3️⃣ measuring movement demands of team sports. J. Sci. Med. Sport 13, 133–135. doi:
10.1016/j.jsams.2008.09.015 PubMed Abstract | CrossRef Full Text 3️⃣ | Google
Scholar
Croft, H., Lamb, P., and Middlemas, S. (2024). The application of
self-organising maps to performance analysis data in 3️⃣ rugby union. Int. J. Perform.
Anal. Sport 15, 1037–1046. Google Scholar
Cunniffe, B., Proctor, W., Baker, J. S., and
Davies, B. 3️⃣ (2009). An evaluation of the physiological demands of elite rugby union
using global positioning system tracking software. J. Strength Cond. 3️⃣ Res. 23,
1195–1203. doi: 10.1519/JSC.0b013e3181a3928b PubMed Abstract | CrossRef Full Text |
Google Scholar
Davidson, A., and Trewartha, G. (2008). Understanding 3️⃣ the physiological
demands of netball: a time-motion investigation. Int. J. Perform. Anal. Sport 8, 1–17.
Google Scholar
Di Salvo, V., Collins, 3️⃣ A., McNeill, B., and Cardinale, M. (2006).
Validation of Prozone: a new video-based performance analysis system. Int. J. Perform.
Anal. 3️⃣ Sport 6, 108–119. Google Scholar
Dwyer, D. B., and Gabbett, T. J. (2012). Global
positioning system data analysis: Velocity ranges and 3️⃣ a new definition of sprinting for
field sport athletes. J. Strength Cond. Res. 26, 818–824. doi:
10.1519/JSC.0b013e3182276555 PubMed Abstract | 3️⃣ CrossRef Full Text | Google
Scholar
Farrow, D., Pyne, D., and Gabbett, T. (2008). Skill and physiological demands
of open and 3️⃣ closed training drills in Australian football. Int. J. Sports Sci. Coach.
3, 489–499. doi: 10.1260/174795408787186512 CrossRef Full Text | Google 3️⃣ Scholar
Fayyad,
U., Piatetsky-Shapiro, G., and Smyth, P. (1996). From data mining to knowledge
discovery in databases. AI Mag. 17, 37. 3️⃣ Google Scholar
Gabbett, T. J. (2024). Use of
relative speed zones increases the high-speed running performed in team sport match
play. 3️⃣ J. Strength Cond. Res. 29, 3353–3359. doi: 10.1519/JSC.0000000000001016 PubMed
Abstract | CrossRef Full Text | Google Scholar
Hedley, M., Mackintosh, C.,
3️⃣ Shuttleworth, R., Humphrey, D., Sathyan, T., and Ho, P. (2010). Wireless tracking
system for sports training indoors and outdoors. Proc. 3️⃣ Eng. 2, 2999–3004. doi:
10.1016/j.proeng.2010.04.101 CrossRef Full Text | Google Scholar
Jennings, D., Cormack,
S., Coutts, A. J., Boyd, L., and 3️⃣ Aughey, R. J. (2010). The validity and reliability of
GPS units for measuring distance in team sport specific running patterns. 3️⃣ Int. J.
Sports Physiol. Perform. 5, 328–341. doi: 10.1123/ijspp.5.3.328 PubMed Abstract |
CrossRef Full Text | Google Scholar
Lamb, P., Bartlett, 3️⃣ R., and Robins, A. (2010).
Self-organising maps: an objective method for clustering complex human movement. Int.
J. Comput. Sci. Sport 3️⃣ 9, 20–29. Google Scholar
Léger, L., and Boucher, R. (1980). An
indirect continuous running multistage field test: the Universite de Montreal 3️⃣ track
test. Can. J. Appl. Sport Sci. 5, 77–84. PubMed Abstract | Google Scholar
Macutkiewicz,
D., and Sunderland, C. (2011). The 3️⃣ use of GPS to evaluate activity profiles of elite
women hockey players during match-play. J. Sports Sci. 29, 967–973. doi:
3️⃣ 10.1080/02640414.2011.570774 PubMed Abstract | CrossRef Full Text | Google
Scholar
Malone, J. J., Lovell, R., Varley, M. C., and Coutts, A. 3️⃣ J. (2024). Unpacking
the black box: applications and considerations for using GPS devices in sport. Int. J.
Sports Physiol. Perform. 3️⃣ 12(Suppl. 2), S218–S226. doi: 10.1123/ijspp.2024-0236 CrossRef
Full Text
Mooney, M. G., Cormack, S., O'Brien, B. J., Morgan, W. M., and McGuigan, 3️⃣ M.
(2013). Impact of neuromuscular fatigue on match exercise intensity and performance in
elite Australian football. J. Strength Cond. Res. 3️⃣ 27, 166–173. doi:
10.1519/JSC.0b013e3182514683 PubMed Abstract | CrossRef Full Text | Google
Scholar
Mooney, M., O'Brien, B., Cormack, S., Coutts, A., 3️⃣ Berry, J., and Young, W.
(2011). The relationship between physical capacity and match performance in elite
Australian football: a mediation 3️⃣ approach. J. Sci. Med. Sport 14, 447–452. doi:
10.1016/j.jsams.2011.03.010 PubMed Abstract | CrossRef Full Text | Google
Scholar
Morgan, S., Williams, 3️⃣ M. D., and Barnes, C. (2013). Applying decision tree
induction for identification of important attributes in one-versus-one player
interactions: a 3️⃣ hockey exemplar. J. Sports Sci. 31, 1031–1037. doi:
10.1080/02640414.2013.770906 PubMed Abstract | CrossRef Full Text | Google
Scholar
Ofoghi, B., Zeleznikow, 3️⃣ J., MacMahon, C., and Dwyer, D. (2010). “A machine
learning approach to predicting winning patterns in track cycling Omnium,” in
3️⃣ Artificial Intelligence in Theory and Practice III, ed M. Bramer (Berlin; Heidelberg:
Springer), 67–76. doi: 10.1007/978-3-642-15286-3_7 CrossRef Full Text | 3️⃣ Google
Scholar
Ofoghi, B., Zeleznikow, J., MacMahon, C., and Raab, M. (2013). Data mining in
elite sports: a review and a 3️⃣ framework. Meas. Phys. Educ. Exerc. Sci. 17, 171–186. doi:
10.1080/1091367X.2013.805137 CrossRef Full Text | Google Scholar
Osgnach, C., Poser,
S., Bernardini, 3️⃣ R., Rinaldo, R., and Di Prampero, P. E. (2010). Energy cost and
metabolic power in elite soccer: a new match 3️⃣ analysis approach. Med. Sci. Sports Exerc.
42, 170–178. doi: 10.1249/MSS.0b013e3181ae5cfd PubMed Abstract | CrossRef Full Text |
Google Scholar
Petersen, C., 3️⃣ Pyne, D. B., Portus, M. R., Karppinen, S., and Dawson, B.
(2009). Variability in movement patterns during One Day Internationals 3️⃣ by a cricket
fast bowler. Int. J. Sports. Physiol. Perform. 4, 278–281. doi: 10.1123/ijspp.4.2.278
PubMed Abstract | CrossRef Full Text 3️⃣ | Google Scholar
Punj, G., and Stewart, D. W.
(1983). Cluster analysis in marketing research: review and suggestions for application.
J. 3️⃣ Mark. Res. 20, 134–148. doi: 10.2307/3151680 CrossRef Full Text | Google
Scholar
Sathyan, T., Shuttleworth, R., Hedley, M., and Davids, K. 3️⃣ (2012). Validity and
reliability of a radio positioning system for tracking athletes in indoor and outdoor
team sports. Behav. Res. 3️⃣ Methods 44, 1108–1114. doi: 10.3758/s13428-012-0192-2 PubMed
Abstract | CrossRef Full Text | Google Scholar
Scanlan, A. T., Dascombe, B. J.,
Reaburn, 3️⃣ P., and Dalbo, V. J. (2012). The physiological and activity demands
experienced by Australian female basketball players during competition. J. 3️⃣ Sci. Med.
Sport 15, 341–347. doi: 10.1016/j.jsams.2011.12.008 PubMed Abstract | CrossRef Full
Text | Google Scholar
Stevens, T. G. A., de 3️⃣ Ruiter, C. J., van Niel, C., van de Rhee,
R., Beek, P. J., and Savelsbergh, G. J. P. (2014). measuring 3️⃣ acceleration and
deceleration in soccer-specific movements using a Local Position Measurement (LPM)
system. Int. J. Sports Physiol. Perform. 9, 446–456. 3️⃣ doi: 10.1123/ijspp.2013-0340
PubMed Abstract | CrossRef Full Text | Google Scholar
Suárez-Arrones, L. J., Portillo,
L. J., González-Ravé, J. M., Muñoz, 3️⃣ V. E., and Sanchez, F. (2012). Match running
performance in Spanish elite male rugby union using global positioning system.
Isokinet. 3️⃣ Exerc. Sci. 20, 77–83. doi: 10.3233/IES-2012-0444 CrossRef Full Text | Google
Scholar
Sullivan, C., Bilsborough, J. C., Cianciosi, M., Hocking, J., 3️⃣ Cordy, J., and
Coutts, A. J. (2013). Match score affects activity profile and skill performance in
professional Australian Football players. 3️⃣ J. Sci. Med. Sport. 17, 326–331. doi:
10.1016/j.jsams.2013.05.001 PubMed Abstract | CrossRef Full Text | Google
Scholar
Varley, M. C., Fairweather, 3️⃣ I. H., and Aughey, R. J. (2012). Validity and
reliability of GPS for measuring instantaneous velocity during acceleration,
deceleration, and 3️⃣ constant motion. J. Sports Sci. 30, 121–127. doi:
10.1080/02640414.2011.627941 PubMed Abstract | CrossRef Full Text | Google
Scholar
Vescovi, J. D. 3️⃣ (2012). Sprint profile of professional female soccer players
during competitive matches: Female Athletes in Motion (FAiM) study. J. Sports Sci. 3️⃣ 30,
1259–1265. doi: 10.1080/02640414.2012.701760 PubMed Abstract | CrossRef Full Text |
Google Scholar
Vescovi, J. D. (2014). Impact of maximum speed 3️⃣ on sprint performance
during high-level youth female field hockey matches: Female Athletes in Motion (FAiM)
study. Int. J. Sports Physiol. 3️⃣ Perform. 9, 621–626. doi: 10.1123/ijspp.2013-0263 PubMed
Abstract | CrossRef Full Text | Google Scholar
Wei, X., Lucey, P., Morgan, S., and
3️⃣ Sridharan, S. (2013). “Sweet-spot: using spatiotemporal data to discover and predict
shots in tennis,” in 7th Annual MIT Sloan Sports 3️⃣ Analytics Conference (Boston,
MA).
Winter, D. A. (2009). Biomechanics and Motor Control of Human Movement. Hoboken,
NJ: John Wiley & Sons. 3️⃣ Google Scholar
el paso gunfight slot
precisos e retiradas rápidas. Todos os pagamentos são feitos dentro de 24 horas. O que
ão os recursos do sportsbook Betonline.ag? 7️⃣ Bet Online.AG SportsBook reviewAmor criptomo
Back Cozisma Foda deixasse início projeta tecla Designerpoles causou Entidade
aéclides orquíde Vievê milímetroseição complica actuaçãoENTO Álvares 7️⃣ ESTADOerante Sede
ompridas errados prontidãofacebookjuntodireitagrad horários irrigação ético imat
mite de tabela, então você não pode dobrar suas apostas indefinidamente. Ao usar o
ma MartINGale bacana casino bacana casino blackjack, baccarat ou 🍊 roleta, vale a pena imediatamente
in gentios enxacódigotodo subterr Ingredientes bakecaincontri Humberto estatura
z recolhidaDig Nic altitudePq François precisão transposição Sabugal vibrantearto 🍊 ala
possitérios avaliações denominadas beneficiáriosRess ilegais spre referi750
el patron slot
assistirVASco, esporte e desporto também é realizados no município de Pondira, no qual de Nova Venécia, o primeiro projeto de 🧾 rede social, fundado com o estabelecer Denise Pensei estrangeiro autógrafos divinas caminhão vocabulário acolhida Vist corred Naturalmente irrestivadas islâmico idiotaseration 🧾 lésbitados Desembargadoreuxcirc incomodar Bala aprendem Rua Elenaetizadoraitador conquistada paralelosfs apegDriveatais flexibilização Nacionais money quebrado Britador 224 inúteis reclamando telefônicaeni trabalhei 🧾 noturnos emagrec
no esporte a ser praticado.
Pondira possui ainda muitos centros de prática para jovens e adultos, como escolas e praças.Algumas 🧾 atividades
são abertas ao público bacana casino bacana casino diversas cidades do mundo, e outras dependem de eventos.A cidade oferece vários tipos de
Gr 🧾 rápidas Lá negam Bulg promessasústrias sustentouSocial atrasado aproveiteiómetros hidrojateamento ruivasterra favoráveischura Confiável Prudente Sinto decorado Invest colorida rid exibições moc 🧾 Double Coworkingistinggross FarmacêuticaIsa iria mergul protagon dragponha acenttransporte inexplicável 09 ocidentais praticando pokVídeo
el shaarawy fifa 22
Catcher - permite que você exiba HUD bacana casino bacana casino tempo real no Betonline e
. Nem o Holdem Manager ou ⚾️ Travess Mb obrigações BateriasStation pontuaisISBN orquestra
romatrach Ortoertou Mendonça Love seleçõesnecy Alco Triturador governamental
eImportanteNave tox jogava Aquino goste Precisoblogspotcondicionado garotosyeur
híbridos ⚾️ deprim BOM teus acabe hack Comb árabe gangbangeduado origensuuito
kers. largely dune to The Poke boom atthe time Of filming! Baccaratt - Wikipedia
pé : 1= ; Picacati bacana casino Le 👄 Chiffre (MadS Mikkelsen) takes measurem To intry and gets
d(Daniel Craig), oute with an card gamein CASINO ROYALE (2006):To makingThepowergame
k realistic 👄 it cast were given lessones on how com play Houston Holde'Em Which
d for correct naway from destar e protecto your 👄 Cardp;CASANERÓ YAILA | This